“We’re really firm believers that by contributing to the community and building upon open-source data models, the whole community moves further, faster,” says Larry Zitnick, the lead researcher for the OMat project.
Zitnick says the newOMat24 model will top the Matbench Discovery leaderboardwhich ranks the best machine-learning models for materials science. Its data set will also be one of the biggest available.
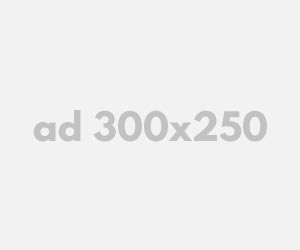
“Materials science is having a machine-learning revolution,” says Shyue Ping Ong, a professor of nanoengineering at the University of California, San Diego, who was not involved in the project.
Previously, scientists were limited to doing very accurate calculations of material properties on very small systems or doing less accurate calculations on very big systems, says Ong. The processes were laborious and expensive. Machine learning has bridged that gap, and AI models allow scientists to perform simulations on combinations of any elements in the periodic table much more quickly and cheaply, he says.
Meta’s decision to make its data set openly available is more significant than the AI model itself, says Gábor Csányi, a professor of molecular modeling at the University of Cambridge, who was not involved in the work.
“This is in stark contrast to other large industry players such as Google and Microsoft, which also recently published competitive-looking models which were trained on equally large but secret data sets,” Csányi says.
To create the OMat24 data set, Meta took an existing one called Alexandria and sampled materials from it. Then they ran various simulations and calculations of different atoms to scale it.
Meta’s data set has around 110 million data points, which is many times larger than earlier ones. Others also don’t necessarily have high-quality data, says Ong.
GIPHY App Key not set. Please check settings