Add the fact that other tech firms, inspired by DeepSeek’s approach, may now start building their own similar low-cost reasoning models, and the outlook for energy consumption is already looking a lot less rosy.
The life cycle of any AI model has two phases: training and inference. Training is the often months-long process in which the model learns from data. The model is then ready for inference, which happens each time anyone in the world asks it something. Both usually take place in data centers, where they require lots of energy to run chips and cool servers.
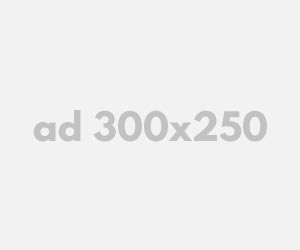
On the training side for its R1 model, DeepSeek’s team improved what’s called a “mixture of experts” technique, in which only a portion of a model’s billions of parameters—the “knobs” a model uses to form better answers—are turned on at a given time during training. More notably, they improved reinforcement learning, where a model’s outputs are scored and then used to make it better. This is often done by human annotators, but the DeepSeek team got good at automating it.
The introduction of a way to make training more efficient might suggest that AI companies will use less energy to bring their AI models to a certain standard. That’s not really how it works, though.
“Because the value of having a more intelligent system is so high,” wrote Anthropic cofounder Dario Amodei on his blog, it “causes companies to spend more, not less, on training models.” If companies get more for their money, they will find it worthwhile to spend more, and therefore use more energy. “The gains in cost efficiency end up entirely devoted to training smarter models, limited only by the company’s financial resources,” he wrote. It’s an example of what’s known as the Jevons paradox.
But that’s been true on the training side as long as the AI race has been going. The energy required for inference is where things get more interesting.
DeepSeek is designed as a reasoning model, which means it’s meant to perform well on things like logic, pattern-finding, math, and other tasks that typical generative AI models struggle with. Reasoning models do this using something called “chain of thought.” It allows the AI model to break its task into parts and work through them in a logical order before coming to its conclusion.
You can see this with DeepSeek. Ask whether it’s okay to lie to protect someone’s feelings, and the model first tackles the question with utilitarianism, weighing the immediate good against the potential future harm. It then considers Kantian ethics, which propose that you should act according to maxims that could be universal laws. It considers these and other nuances before sharing its conclusion. (It finds that lying is “generally acceptable in situations where kindness and prevention of harm are paramount, yet nuanced with no universal solution,” if you’re curious.)
GIPHY App Key not set. Please check settings