Over the past 12 months, customer conversations have shifted from focusing on generative AI to discussing agentic AI. This evolution reflects the growing recognition of agentic systems to augment AI’s potential to enhance business processes and drive innovation.
But, as with every technology, working out where to start is fraught with difficulties. “When all you have is a hammer, everything looks like a nail”—or so the expression goes—but when it comes to business challenges, not every problem warrants an agentic AI approach.
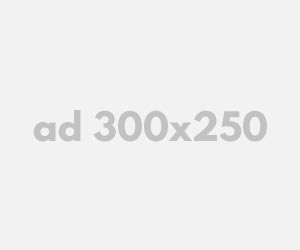
You may have determined candidate areas for agentic AI using a similar approach to that which we described when discussing rapidly ideating on value in a previous blog. However, how do you know if it really warrants an agentic approach, and then, once you’re confident that it does, how do you determine the value it will bring for your organization?
This blog aims to provide guidance on how to address these areas to empower you to make informed decisions and unlock the full potential of agentic AI.
Business and technical criteria
Based on our experience working with retail and consumer goods companies across the globe, there are some common trends that can be considered as criteria for determining if a specific process—or part of a process—is a good use case for agentic AI.
These aren’t considered to be “hard and fast” criteria that must be adhered to—they are merely guidelines.
Volume. A process with high volumes or number of interactions. For example, a consumer goods company receives many more orders than an aircraft manufacturer, therefore, it’s likely to be far more applicable to apply agentic AI to an order intake process in a consumer goods company. That doesn’t mean that agentic AI cannot help an aircraft manufacturer with this process. It means that the specific process element where it’s applied would be different. For example, in placing an order for an aircraft, multiple detailed configuration documents may be needed, and agentic AI may have a valuable role ensuring those documents are correct.
Interaction. A process that interacts with multiple systems. For example, updates, reads from, or consolidates data between different systems. Processes where users must review, or consolidate, content from multiple systems are prime candidates for the application of agentic AI. Sometimes referred to as “swivel-chair integration,” these types of processes are both tedious and fraught with error.
Human. A process where a high level of human interaction is required. Perhaps involving seeking, reading, considering, and reasoning over multiple pieces of information, documents, or systems. This is typically work that’s mundane and repetitive. Agentic AI can assess and highlight gaps, differences, or anomalies. It can make recommendations to be evaluated by a human and as such, is designed to work alongside or augment the human by reducing the amount of mundane, repetitive activity. The human element is critical here—AI allows the human to focus on exceptions, strategic analysis, and complex decisions while supporting innovation.
Errors. Processes that are error prone—which often occurs with repetitive, mundane human operations. More importantly, one where any errors or issues during the process execution cause adverse downstream consequences such as delayed deliveries, lost sales, compensation claims, or handling by a human that incurs cost or time. This can be a key area of concern and focus.
There is an additional requirement, albeit one that must be considered when architecting a solution. This relates to data availability.
It’s critical to ensure that the data required for the agentic AI application is available and accessible without causing challenges elsewhere. It’s common that agentic systems need to refer to data to aid decision-making. For example, it may be necessary to look something up on a customer or supplier master record in a transactional system. Where many of these are required in a very short time, it may be that the agentic solution causes performance issues in the transactional system. Architecturally, this challenge can be avoided by extracting this data into a data lake or other data store to act as a reference location.
Retail Thought Leadership Study
The AI Advantage: How retailers are shaping customer experiences with data-driven insights
Defining value
Advancements position agentic AI as a cornerstone for creating a more resilient, efficient, sustainable, and autonomous supply chain. When it comes to evaluating the business value of any technology investment, one of the first points to consider is determining the specific drivers of value. In addition, understanding how you’ll measure this is equally important.
From the work we have done relating to agentic AI, value typically falls into three areas:
Productivity. You can think of this as “agentic liberated time.” This reflects reducing the non-value-added time associated with human interaction in a process or process step using the “liberated time” for value-added activities. Scoping these additional activities is critical to delivering value from agentic AI. As an example, one retailer was seeking to free up time for their supply chain planners to spend more time with individual suppliers planning future promotional inventories. AI agents can streamline communications with suppliers, monitor contract compliance, and resolve disputes efficiently.
Process efficiency. This relates to the elapsed time that a process takes. AI agents automate repetitive tasks and optimize operations leading to higher process efficiency levels and lower costs. This in turn has follow-on benefits—for example, reducing the time spent between receiving and processing a customer order translates to improved customer responsiveness.
Quality. This can often be seen as cliché. However, in this instance, the focus is the reduction of errors or issues. Specifically, those that have a negative consequence downstream within the organization or supply chain. For example, promising inventory that does not exist will adversely impact customer satisfaction scores and may well result in future lost sales.
Measurement is key
For each of these value driver areas it’s important to establish the metrics or KPIs that this is likely to impact in your specific case. The graphic above gives some examples, but this is where the value of agentic AI really comes into force.
For the productivity value driver, liberated time can be used to identify additional revenue generating opportunities, which can enhance your revenue per employee KPI. For process efficiency, reducing lost sales can be a relevant metric if, for example, you’re automating your customer order process.
Quality, however, is where it becomes interesting. Determining the downstream negative consequences of a delayed or misinformed decision can be difficult, but it’s worthwhile. One approach to consider is to use Microsoft Copilot to help ideate on this, asking for suggestions as to what the negative downstream consequences of errors in a particular process might be. This may not yield the exact answer for your business, but practice has shown that it usually inspires a new thought or perspective that relates to your business.
Microsoft Cloud for Retail
Connect your customers, your people, and your data.
Moving on value
Selecting the right use cases for agentic AI requires a thorough understanding of both the criteria for implementation and the drivers of value. By focusing on high-volume, error-prone processes that require significant human effort and interaction with multiple systems, organizations can identify the most promising areas for AI application.
Additionally, defining and measuring the value of AI investments through productivity, process efficiency, and quality improvements will ensure that organizations can unlock the full potential of agentic AI. With these guidelines, organizations can make informed decisions and navigate the complexities of AI use case selection, ultimately driving innovation and efficiency.
Learn more about agentic AI
Oliver Guy
Global Industry Architect, Microsoft Retail & Consumer Goods, Microsoft
Oliver Guy, Global Industry Architect, specializes in helping business leaders innovate and compete more flexibility and efficiently. For more than 25 years he has delivered value for retail and consumer goods companies across the globe with technology led change. Oliver is a recognized Retail Technology Influencer by Retail Technology Innovation Hub (RTIH) and is also a RetailWire BrainTrust panelist.
See more articles from this author
Felice Miller
Business Strategy Leader, Supply Chain & Operations, Worldwide Retail and Consumer Goods and Gaming, Microsoft
Felice leads Supply Chain and Sustainability Strategy for Microsoft’s Worldwide Retail and Consumer Goods Industry Group collaborating with customers and partners to reimagine data and AI solutions, and leveraging technology to drive innovation and better business outcomes. She has extensive experience in consumer products, retail, and global manufacturing. Felice has been an angel investor in early-stage startups, the founder of Delvv, a machine learning studio that created AI-driven interface technology to enhance the smartphone user experience and is an advocate for consumer-centric technology in the mobile space.
See more articles from this author
Paul cheat
Principal Industry Architect, Industry Solutions Delivery, Microsoft
As a Manufacturing industry architect, Paul works with senior business and IT leaders to help them understand how to apply Microsoft’s technologies and partner solutions to digitally transform their company. Leveraging over 35 years of manufacturing industry experience, Paul works with clients to build their transformations strategy, considering four key pillars of digital transformation: customer experience, operational excellence, workforce transformation and product-as-a-service.
See more articles from this author
Source link
GIPHY App Key not set. Please check settings